The healthcare industry is nervous about Artificial Intelligence (AI). A June 2024 BCG survey found that healthcare ranks lowest in confidence and highest in anxiety when it comes to AI integration. While this caution is understandable given the stakes involved, it’s increasingly clear that AI isn’t just another tech trend. It’s a game-changer that’s already revolutionizing how we diagnose diseases, develop drugs, and handle the mountain of paperwork that’s drowning our healthcare professionals.
The Promise of AI in Healthcare
Enhanced Diagnostic Accuracy
The journey of AI in medical diagnosis began in the 1970s with MYCIN, an AI program designed to identify blood infections. Today, machine learning algorithms have evolved to demonstrate remarkable success in disease diagnosis, achieving accuracy rates around 90% for a wide range of conditions, from Alzheimer’s to breast cancer. The synergy between AI and human expertise has proven particularly powerful with studies showing that when physicians are aided by deep learning algorithms, their ability to identify cancerous cells increases from an already impressive 96% to an outstanding 99.5%.
In a groundbreaking development, Google’s Articulate Medical Intelligence Explorer (AMIE) achieved a significant milestone in 2024 by actually outperforming a panel of doctors in patient diagnosis through conversational evaluation, utilizing advanced Natural Language Processing and Machine Learning (ML) techniques.
Drug Development Revolution
The pharmaceutical industry faces a significant challenge: over 90% of protein-based drug candidates fail in clinical trials, with each failure costing between $30 and $300 million. AI is reshaping this landscape. The technology’s ability to generate and test novel protein combinations before physical synthesis is proving invaluable. Major pharmaceutical companies are taking notice—Eli Lilly and Novartis have partnered with Alphabet to develop AI-powered drug discovery platforms, using algorithms trained on domain-specific data to select for specific properties and interactions.
Administrative Efficiency
The burden of administrative tasks weighs heavily on healthcare professionals, who currently spend more than twice as much time on paperwork as they do with patients. Many even dedicate an additional one to two hours of personal time each night to data entry. AI offers a solution to this imbalance by automating numerous tasks. Imagine a system that prepares patient summaries before sessions, seamlessly translates clinical notes into Electronic Health Records (EHR) systems, generates referrals, and handles insurance filings and hospital admittance paperwork. Beyond these day-to-day tasks, AI can also keep healthcare providers updated on the latest findings in their field, ensuring they stay at the forefront of medical advancements.
Implementation Considerations
The Regulatory Landscape
As of June 2024, the regulatory environment for AI in healthcare remains complex. While the FDA has authorized 882 AI/ML-enabled medical devices, there are no formal requirements specifically for AI-enabled devices. Instead, the agency has published general guidelines and proposed frameworks, expressing particular concern about applications that may change themselves over time—a fundamental characteristic of many AI systems.
Organizations must navigate this landscape using two primary reference documents: the Predetermined Change Control Plan and Good Machine Learning Practice for Medical Device Development.
- Leveraging multi-disciplinary expertise throughout the total product lifecycle
- Implementing good software engineering and security practices
- Ensuring clinical study participants and data sets represent the intended patient population
- Maintaining independence between training and test data sets
- Selecting reference datasets based on best available methods
- Tailoring model design to available data and intended use
- Focusing on the performance of the human-AI team
- Testing device performance during clinically relevant conditions
- Providing users with clear, essential information
- Monitoring deployed models for performance and managing re-training risks
Adding to the complexity, various states have enacted their own regulatory frameworks, creating a multilayered compliance challenge that forces companies to navigate a tangled web of shifting requirements. This regulatory uncertainty can significantly impact implementation timelines and costs.
Despite these challenges, the regulatory landscape isn’t intended to prevent innovation, but rather to ensure patient safety and effective outcomes. Organizations that understand and proactively address these regulatory considerations can more effectively navigate the path to AI implementation.
Legal Considerations
The legal landscape surrounding AI in healthcare is equally intricate. Most lawsuits to date have centered on health insurance companies, with patients claiming wrongful denial of coverage using AI. A notable example is the lawsuit against United Healthcare, which alleges the company denied claims despite knowing that approximately 90% of their tools’ denials were faulty. Physicians face their own legal vulnerabilities—they may be subject to malpractice suits regardless of whether they follow AI advice. Algorithm developers aren’t immune either, potentially facing liability for injuries resulting from poor design or failure to warn about risks.
Key Concerns and Mitigation Strategies
The implementation of AI in healthcare faces several significant challenges. Model bias remains a critical concern—a 2019 study revealed that an algorithm used to predict healthcare needs showed bias against Black patients due to historical inequities in healthcare access. The “black box” effect, where an algorithm’s decision-making path cannot be traced, also poses significant challenges in healthcare’s strictly regulated environment. Data monopolies present another worry, as larger medical providers with more data may strengthen their positions, leading to anti-competitive practices. In response to this last concern, the DOJ launched the Task Force on Health Care Monopolies and Collusion in March 2024.
Strategic Recommendations
Quality Management Systems
In the development of new drugs and healthcare technologies, many organizations implement Quality Management Systems (QMS) to ensure product efficacy and safety. A significant challenge they face is the effective integration of AI products into these existing QMS frameworks. Fundamentally, organizations should treat AI systems similarly to other complex and high-risk clinical processes, such as those in radiation oncology. Mapping out the entire process can help define the likelihood of failures at each step and understand how these failures may impact and propagate throughout the system. This approach should also include clear procedures for mitigating identified issues. Finally, it is crucial to provide users with the necessary knowledge to operate these products safely while also making them aware of their shared responsibilities in the operation of the technology.
Human-in-the-Loop Approach
The Human-in-the-Loop (HITL) approach integrates human expertise into the decision-making processes of AI systems, leveraging the strengths of both machines and humans. AI systems excel at processing large volumes of data and recognizing patterns, while human clinicians contribute critical thinking, empathy, and nuanced understanding. Current FDA guidance advocates for HITL systems to maximize the safety and effectiveness of new medical devices for their intended users and environments. Effective HITL implementation involves several phases: preparation, development, and validation. During preparation, organizations should define appropriate training data and ensure it comprehensively represents the patient base. The development phase allows for rapid iteration and modification of AI algorithms, validating outcomes in both development and early clinical trials. Finally, AI decisions must always be validated by experts before being delivered to patients, ensuring that AI complements rather than replaces professional judgment.
Explainable Artificial Intelligence (XAI)
Explainable AI (XAI) seeks to clarify the reasoning behind the decisions made by deep learning models, often characterized as “black boxes.” XAI can be categorized into two types: ante-hoc, which is integrated into existing models, and post-hoc, which operates independently of the models. In healthcare, post-hoc strategies are often preferred due to their versatility across various models. The importance of XAI in healthcare is manifold. It builds physician trust through model interpretability, empowers patients by offering multiple options, and aids in uncovering hidden biases within algorithms. Additionally, XAI contributes to debugging and improving AI systems while fulfilling QMS and regulatory requirements. Notable XAI algorithms include Grad-CAM, Local Interpretable Model-Agnostic Explanations, and Shapley Additive Explanations, each designed to enhance understanding of AI decision-making processes.
Open Source in Healthcare AI
The adoption of open-source AI technologies has the potential to significantly improve global health outcomes. Open source plays a critical role in addressing the distrust among clinicians that stems from a lack of transparency regarding model design and data origins. By enabling shared diagnostic data, open-source frameworks enhance the capabilities of AI models and promote standardization, innovation, and competition within the field. Furthermore, open-source initiatives help prevent data monopolies, lower barriers for healthcare providers with limited resources, and ensure equitable access to healthcare technologies regardless of race, gender, or wealth. Notable open-source AI projects in healthcare include Seismometer for AI accuracy validation, ChRIS for image processing in radiology, MONAI for deep learning image analysis, and the Hippo AI Foundation’s generalized framework.
Transparency
In general, transparency is paramount; providing easy ways for physicians and patients to compare raw data with AI-generated results allows for effective supervision. Clear communication regarding how the AI process works and the management of patient data is essential for building trust. Additionally, configurations should allow adjustments based on physician and patient comfort, with contingency plans in place should either party choose to opt out of AI involvement.
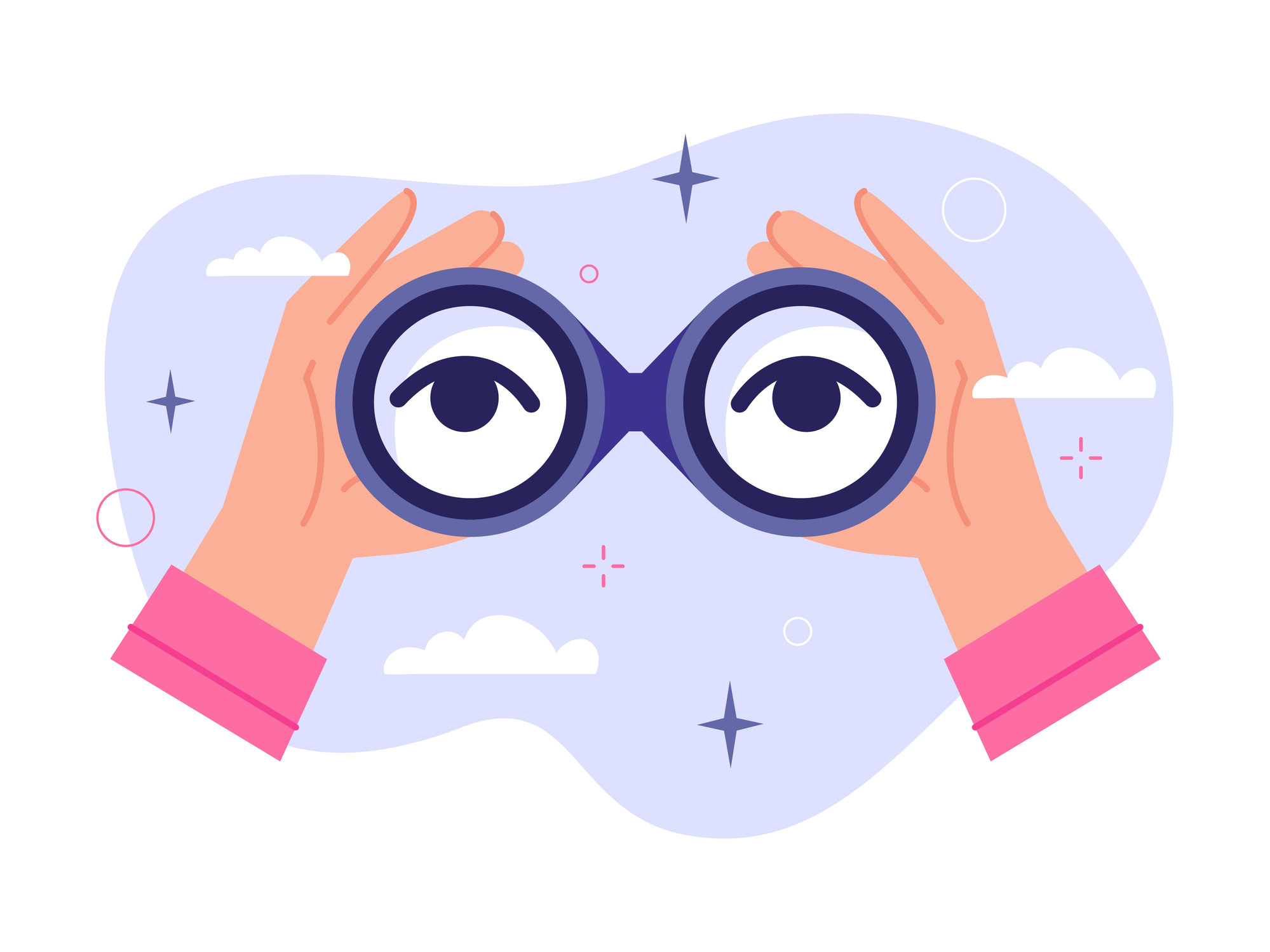
Looking Ahead
Due to the novelty of these new AI technologies, in addition to the regulations and expectations inherent to the healthcare environment, we can expect these trends to shape the AI landscape in the near future:
- Pre-Built Models and Tools: The availability of vetted AI tools, such as AWS HealthScribe and AMIE, will facilitate faster integration into healthcare systems without necessitating extensive ML infrastructure.
- Low-Risk Implementations: Generative AI is likely to find its most effective applications in low-risk environments, where historical data can guide decision-making. This cautious approach allows healthcare providers to build trust in AI capabilities.
- Complementarity Over Replacement: While discussions of Artificial General Intelligence continue, for now the focus will likely remain on AI complementing existing workflows, enhancing efficiency without undermining the human element of care.
Key Implementation questions
For healthcare organizations considering AI integration, several critical questions must be addressed: How do the benefits weigh against the technical and regulatory complexity? What are the true costs, including not just the technology but also staffing, data storage, and ongoing maintenance? How will AI systems integrate with existing quality management protocols? What legal implications must be considered, particularly regarding data privacy and security? And perhaps most importantly, how can organizations ensure transparency and maintain patient trust through proper consent procedures?
While integrating AI into healthcare systems presents significant challenges, the potential benefits—from improved diagnostic accuracy to more efficient drug development and streamlined administrative processes—make it a journey worth undertaking. By approaching AI integration strategically, with a focus on compliance, quality management, and transparency, healthcare organizations can harness the power of AI while maintaining their commitment to patient safety and care quality. The future of healthcare lies not in choosing between human expertise and artificial intelligence, but in finding the optimal way to combine both.
Let’s Talk
Reach out to us so we can help you, whether you have a new healthcare product need, a new version of an existing product or want to integrate a new technology.
Contact Us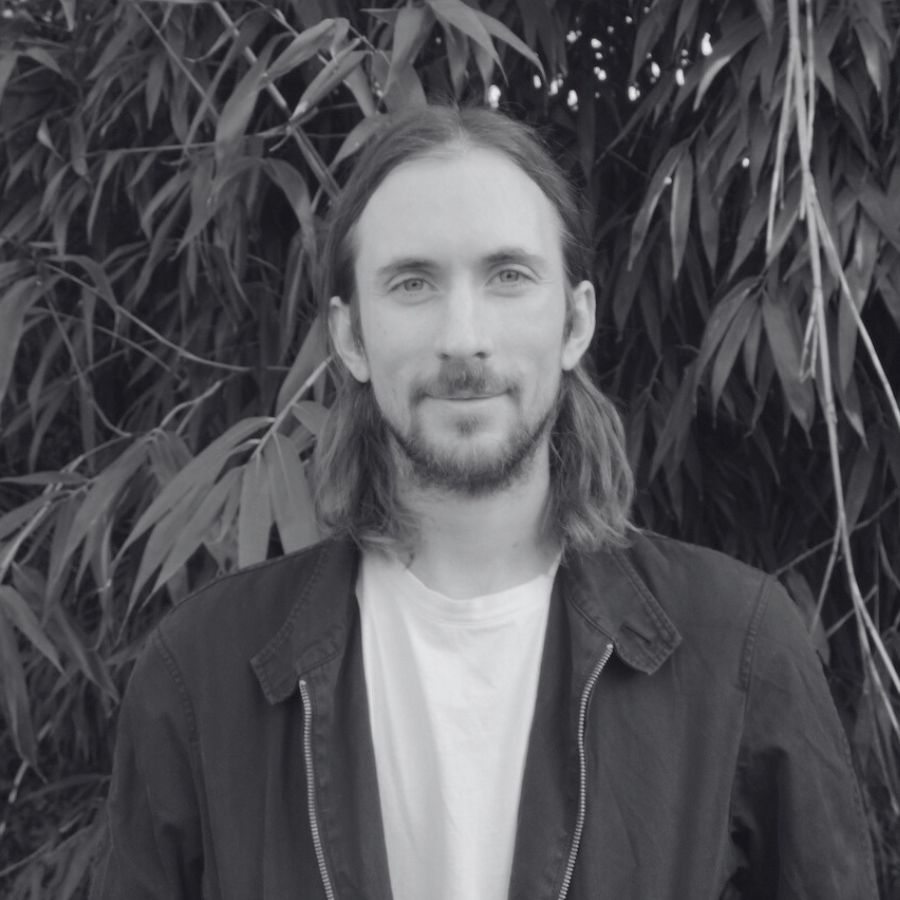
Steven Davis is a fullstack developer with over 10 years experience building software for the web and mobile applications. He has 4+ years experience working as a consultant and engineering lead on projects for healthcare and life science companies, and is currently a Manager in Product Engineering here at Dialexa.